Frameworks, Frameworks, ...
- Tamara Polajnar
- Feb 27, 2024
- 6 min read
...Frameworks: Ethical AI frameworks, why are there so many? Why did we add another?
IF you look for them, you will dig them up. Ethical Artificial Intelligence (AI) frameworks are somewhere out there, but is anyone using them?
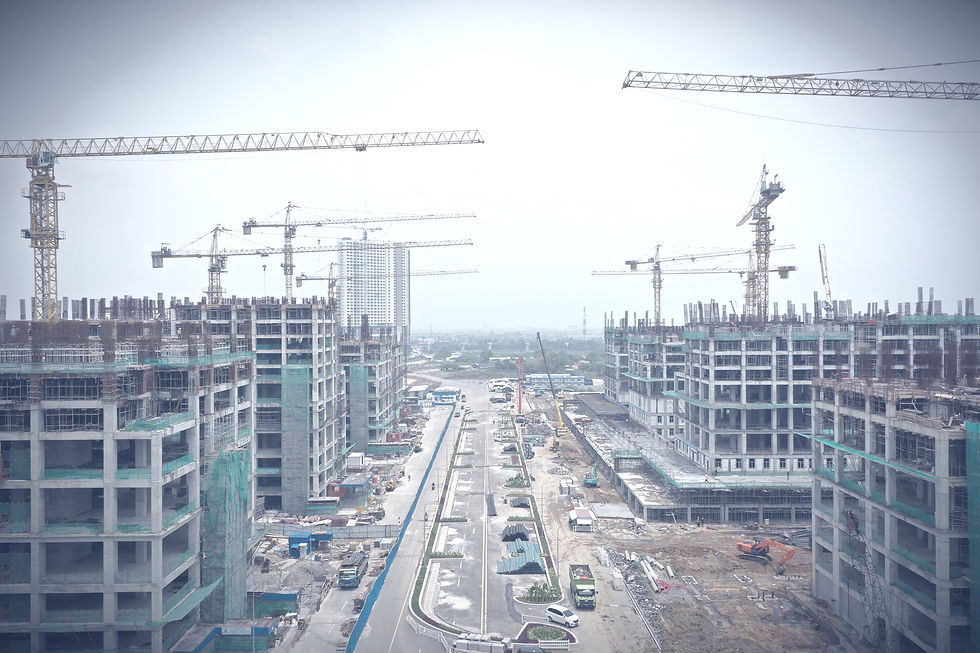
The UK government alone has the Algorithmic Transparency Recording Standard (ATRS), Data Ethics Framework, and the Information Commissioner's Office (ICO) guidelines, toolkit, and a guide for auditing AI applications. There are more in the US, including ones by NIST and the military. Certainly, every country will have their own versions in the near future.
Machine learning advances that are leaving experts confounded are increasing demands for control, regulation, and understanding, leading to proliferation of advice. The advice as such is not conflicting or unique but presented from different perspectives and for different audiences. At the core of all of the frameworks is the call for thoughtful development of AI:
Making sure the problem that is being solved is clearly defined and contained.
Understanding and clearly documenting the data that is being fed into the model, how it was produced, and what are the inevitable encoded biases in this data.
Choice of model and bias mitigation at the development stage.
Recording of all decisions that impact the model performance and could also introduce bias.
Deployment of the model in a way that includes human oversight, monitors the model performance and potential harmful behaviour, and clearly matches with the initial design goals.
Ethical frameworks for policing and beyond
Public organisations whose decisions affect our lives need to ensure as much as possible they are mindful in their application of automation. As the tech giants and crafty startups race for the holy grail of the best AI with little oversight, we wield humble frameworks, and hope to encourage ethical development on the application level.
The questions are then:
And finally...
Are the ethical AI frameworks being used, and how?
That is a hard question to answer, but indications are that, at the moment, there is no standard framework that the police forces are embracing across the board although they are doing internal ethical reviews, have internal documentation policies, and some have adapted ALGOCARE guidelines for their own purposes. Certainly, there are very few published reports on the ATRS registry and the review paper has flagged up low uptake due to several concerns including "misperception of the dangers of policing technology, and a worry that the Standard will become an administrative burden rather than a benefit for policing or the public". Both of which are concerns easily translated to other frameworks.
Furthermore, as ATRS also encourages public posting of algorithm details there is an added concern for policing and security services about reporting full details of their algorithms, although this is not required.
Is there one framework to rule them all, at least for policing?
The ICO toolkit is mainly concerned with the data processing, storage, and data rights of the individuals in the dataset, although it does touch aspects of bias and accuracy. It is very much formulated as a series of yes or no questions. For example,
Have you considered how you will prevent bias throughout the project?
The ATRS does ask longer questions, and has a format similar to a combination of data and model cards. For example, the guideline for the model maintenance documentation is:
The attribute 'maintenance' gives details on the maintenance schedule and frequency of any reviews. This includes information such as how often and in what way the tool is being reviewed post-deployment, and how it is being maintained if further development is needed. This can concern maintenance both by the supplier as well as by the operator/user of the tool.
However, while most police forces have analytics teams they do not have a lot of institutional understanding or experience in machine learning (ML). This means that when it comes to development or even procurement of ML-based products there is a lack of knowledge regarding which steps are required and what are the key ways to ensure ethical development.
Therefore frameworks that only ask what steps were completed after the fact require completion by a knowledgeable data scientist to ensure that all the steps that are implied in a general question are addressed adequately. What does it really mean to consider bias throughout the project? What does it mean in the context of policing, specifically?
There is a framework that I am yet to mention, that is specific to policing: the INTERPOL Artificial Intelligence Toolkit. It is a beautiful piece of work which carefully considers what is required by a police force that is at the beginning of their responsible AI Journey. It sets out definitions and explains what an organisation needs to do holistically, because ethical AI requires buy-in from the very top to the very bottom, and most importantly from the community which is going to be affected by the changes in future policing practices. The only downside is the fact that the framework is presented as a set of static PDF documents, and therefore the information is difficult to navigate.
Our approach: RUDI
On this site we introduce yet another framework, designed with police in mind. Our framework is less detailed in some of the introductory aspects of responsible AI than the INTERPOL one but offers step-by-step practical guidance from the conceptualisation phase through to implementation, in a more structured way. That is why RUDI, which stands for Rationale, Unification, Development, and Implementation, complements the INTERPOL AI toolkit.
We intend this to be a collaborative site with input from police forces, a living document that can respond to the needs of people at the forefront of responsible AI development in law enforcement. While you will still need to keep up to date with the data protection legislation, filling out the ICO questionnaires and transparency reports should be more straight-forward if you follow the steps outlined in RUDI.
At present RUDI does not have guidance for procuring external AI products, other than to request transparency and detailed descriptions that include notes on ethical considerations as well as model and data cards from any vendors. Ideally, they would be able to provide a satisfactory version of RUDI or ATRS, as that would ensure that the force can be responsible towards its community.
We do advocate that, where there are skills and means, police develop their own algorithms and foster a culture of machine learning literacy within, as this can not only benefit other operations, but ensure that any models they develop are fully within their own control and can be run or cancelled without considerations for costly licence fees.
It's still more paperwork? Why me?
And yes, RUDI like other frameworks is still paperwork-based. This is because the only way to ensure that you have thought through all the important aspects and that there is an institutional memory about the processes is that it is all recorded.
Since algorithms that can affect members of the public are subject to laws that allow people to challenge decisions that can affect them adversely, clear documentation can help protect the organisation.
A key advice in our framework is that responsible AI is implemented institutionally through a multidisciplinary team lead by a Senior Reporting Officer. By separating RUDI into clear sections with documentation supporting each step of the project lifecycle, we distribute the work that goes into documentation across the team instead of needing to rely on the developer.
Most ML projects will start off as a proof of concept and in this case, it might be useful to keep a light version of the Development section going as a set of notes.
Bringing in various colleagues from active-duty officers to senior leadership to work through conceptualisation will help determine whether a prototype is something that can help the organisation in the right way. Similar process can be followed when the driver for development is an organisational need rather than a prototype.
Then once there is an agreement that a prototype should become a product, especially a public-facing one, RUDI can help coordinate communication until deployment and maintenance are established. It may be necessary to revisit every decision in development, as one should not rely on a prototype as the final product.
Frameworks, the necessary... good
So, finally, there are many ethical AI frameworks out there. Draw on them, learn from them, and definitely use them to help guide you through the right questions while you are considering automating internal processes and key decision-making using ML-based solutions.
Kommentare